Real or fake text? We can train ourselves to recognise the distinction
As educators reevaluate learning in the wake of ChatGPT, concerns about the integrity of the job market have spread to the classroom from the creative economy to the managerial class.
Despite the fact that concerns about employment and education dominate the news, the effects of large-scale language models such as ChatGPT will affect virtually every aspect of our lives. These new tools raise societal concerns regarding the role of artificial intelligence in reinforcing social biases, committing fraud and identity theft, creating fake news and disseminating misinformation, among other things.
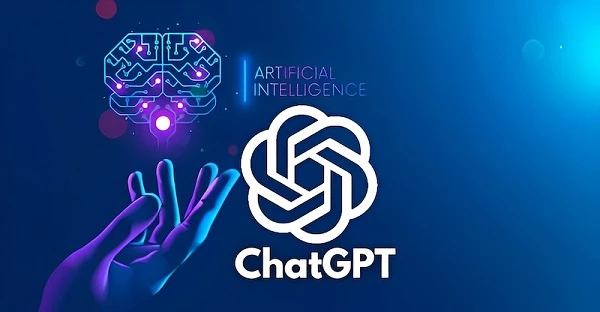
The effects of large-scale language models such as ChatGPT will affect virtually every aspect of our lives. (Illustrative image).
To enable tech users to lessen these risks, a group of researchers at the University of Pennsylvania School of Engineering and Applied Science is working. The authors demonstrate that people can learn to distinguish between machine-generated and human-written text in a peer-reviewed paper presented at the Association for the Advancement of Artificial Intelligence meeting in February 2023.
It's crucial to be aware of the steps you can take to evaluate the credibility of your source before selecting a recipe, sharing an article, or giving out your credit card information.
Chris Callison-Burch, Associate Professor in the Department of Computer and Information Science (CIS), and Ph.D. students Liam Dugan and Daphne Ippolito provide evidence that AI-generated text is detectable in their study.
Callison-Burch states, "We have demonstrated that people can train themselves to recognise machine-generated texts." People make certain assumptions about the types of errors a machine might make, but these assumptions are not always accurate. Given sufficient examples and explicit instruction, we can learn to recognise the types of errors that machines currently commit over time.
Dugan adds, "AI today is surprisingly proficient at producing extremely fluent and grammatically correct text." "But it does make mistakes. We demonstrate that machines make distinct types of errors that we can learn to recognize, such as common-sense errors, relevance errors, reasoning errors, and logical errors.
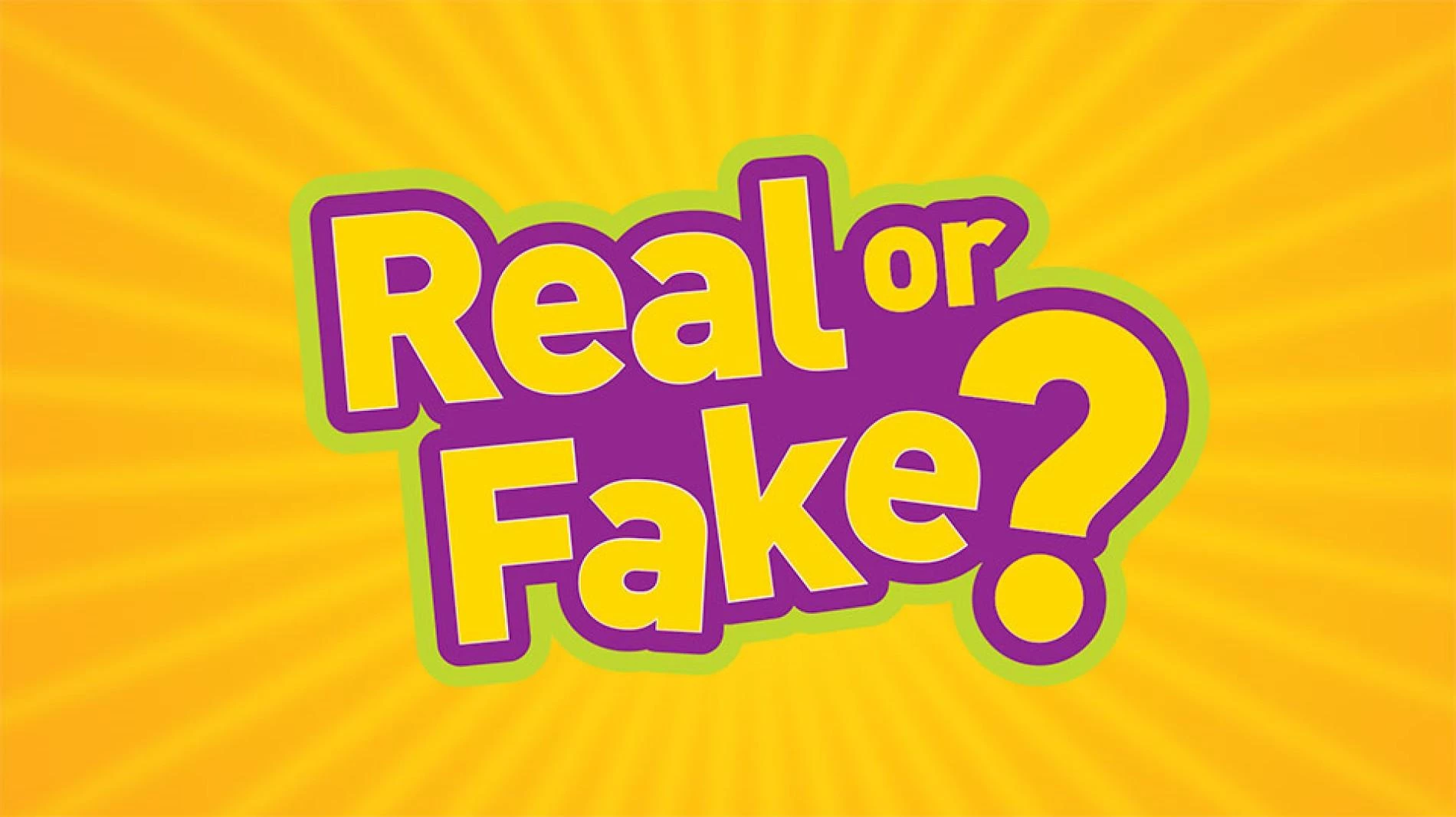
The research utilises data collected from Real or Fake Text?, an original online training game. (Illustrative image).
With the help of this training game, detection studies' usual experimental approach has been transformed into a more realistic simulation of how real people use artificial intelligence to produce text.
Standard methods require participants to indicate whether a machine produced a given text with a yes-or-no response. This task entails classifying a text as authentic or fabricated, and responses are scored as correct or incorrect.
The Penn model refines the standard detection study into an effective training task by displaying examples that are all initially written by humans. Each example is followed by a transition to generated text, and participants are asked to indicate where they believe the transition begins. The trainees identify and describe the textual characteristics that indicate an error, and then receive a grade.
The results of the study indicate that participants performed significantly better than chance, providing evidence that AI-generated text can be detected to some extent.
Dugan explains, "Our method not only gamifies the task, making it more engaging, but it also provides a more realistic training context." "Generated texts, such as those generated by ChatGPT, start with human-supplied prompts."
The study describes not only the current state of artificial intelligence, but also a promising and even exciting future for our relationship with this technology.
Five years ago, according to Dugan, models struggled to stay on topic and construct coherent sentences. Now, they rarely commit grammar errors. Our research identifies the types of errors that are typical of AI chatbots, but it is essential to remember that these errors have evolved and will continue to evolve. The change to be concerned about is not the indetectability of AI-written text. People will need to continue to train themselves to distinguish between the two and use detection software as a supplement."
"People are concerned about AI for legitimate reasons," says Callison-Burch. "Our research provides evidence to alleviate these concerns. Once we are able to harness our optimism regarding AI text generators, we will be able to focus on the capacity of these tools to assist us in writing more imaginative and engaging texts."
Ippolito, co-leader of the Penn study and current Research Scientist at Google, complements Dugan's emphasis on detection by examining the most effective use cases for these tools. She contributed, for instance, to Wordcraft, an AI tool for creative writing developed alongside published authors. None of the writers or researchers viewed artificial intelligence as a convincing substitute for a fiction writer, but they did find significant value in its ability to facilitate the creative process.
Callison-Burch believes that these technologies are best suited for creative writing at this time. "News articles, term papers, and legal advice are poor use cases because there is no factual guarantee."
Dugan states: "There are exciting positive directions you can push this technology in." People are fixated on troubling examples, such as plagiarism and fake news, but we now know we can train ourselves to become better readers and writers.
End of content
Không có tin nào tiếp theo